《Feature Selection and Data-Driven Model for Predicting the Remaining Useful Life of Lithium-Ion Batteries》
In order to ensure the long-term reliable operation of energy storage workstations, accurate, fast and stable remaining life prediction of lithium-ion batteries has become a hot research topic nowadays. In this work, we study the discharge capacity-voltage curve and maximum discharge capacity-cycle curve of lithium batteries, train the short-term model using convolutional-long short-term memory (CNN-LSTM) neural network, and train the long-term iterative model using long short-term memory (LSTM) neural network, and finally obtain a very accurate estimate of the remaining battery life and subsequent changes in the discharge capacity in the early stage of the life of lithium batteries. In this work, the health index output from the short-term model well links the two kinds of neural network models, and the advantages of iterative prediction and short-term prediction are integrated through two different training methods, and finally an excellent performance with an average prediction error of less than 5% is achieved in the MIT dataset. Based on the above model, this work designs a prediction process framework suitable for practical applications, through which the fused long and short-term models mentioned in this work can be quickly deployed in practice. This work illustrates the broad prospects of both feature selection and data-driven methods in the field of remaining life prediction of lithium-ion batteries in a wide cyclic dataset, which provides a certain reference for the future multivariate condition monitoring of batteries.
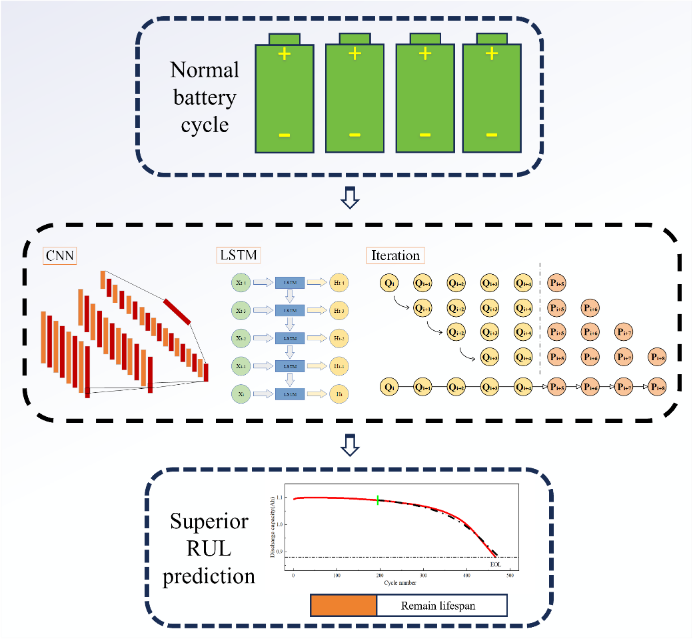
https://ietresearch.onlinelibrary.wiley.com/doi/full/10.1049/esi2.12171